The Current Role of Artificial Intelligence in the Field of Headache Disorders, with a Focus on Migraine: A Systemic Review
Article information
Abstract
The application of artificial intelligence (AI) in the field of headache disorders, particularly migraine, is rapidly expanding, and AI has demonstrated significant potential for diagnosis, treatment, and research. This review examines the current role of AI in migraine management, categorizing AI applications into diagnosis and classification, assessment of treatment response, prediction of migraine attacks, and research. A systematic review of literature published between 2000 and 2024 was conducted, following PRISMA guidelines and utilizing the snowball technique. Of the 398 articles identified, along with five additional articles, 61 were finally reviewed. The results highlight promising AI applications, including the use of patient questionnaires, natural language processing, and imaging for migraine diagnosis, as well as predicting treatment responses and forecasting migraine attacks. Nonetheless, challenges remain in improving the accuracy, generalizability, validation, and clinical relevance of AI applications. Despite the substantial promise of AI for improving migraine management, it does not always guarantee better results than traditional methods. Careful consideration of the study design and method selection is crucial. Additionally, the interpretation of AI-generated results, particularly those from generative models, requires caution to avoid potential pitfalls.
INTRODUCTION
The evolution of artificial intelligence (AI) has been transformative, significantly impacting various aspects of the medicine, including diagnosis, treatment, research, and the development of medical devices. However, the application of AI in the field of headache disorders, including migraine, has been relatively slow. A meta-analysis published in 2020 revealed that only four (<1%) of the 985 selected articles published on Google Scholar between 2010 and 2019 that utilized deep learning (DL) techniques focused on migraine. In contrast, 303 (40%), 161 (21%), and 131 (18%) of these articles addressed Alzheimer’s disease, autism, and epilepsy, respectively.1 Nevertheless, research, tools, and applications related to migraine and headache disorders have expanded considerably since then, leading to a significant increase in published studies. According to a systematic review of computerized migraine diagnostic tools, the number of such tools has increased by 4.5 times since 2005, compared to the period before 2005.2
The current concept of AI and its application in the field of headache disorders is summarized in Figure 1. Briefly, AI can be categorized into symbolic and statistical methods. The symbolic method is based on logic and rule-based reasoning, using knowledge as inputs to produce knowledge that can be directly interpreted.3 Statistical methods generally rely on raw, continuous inputs and use statistical techniques to produce associations that need to be interpreted with background knowledge.
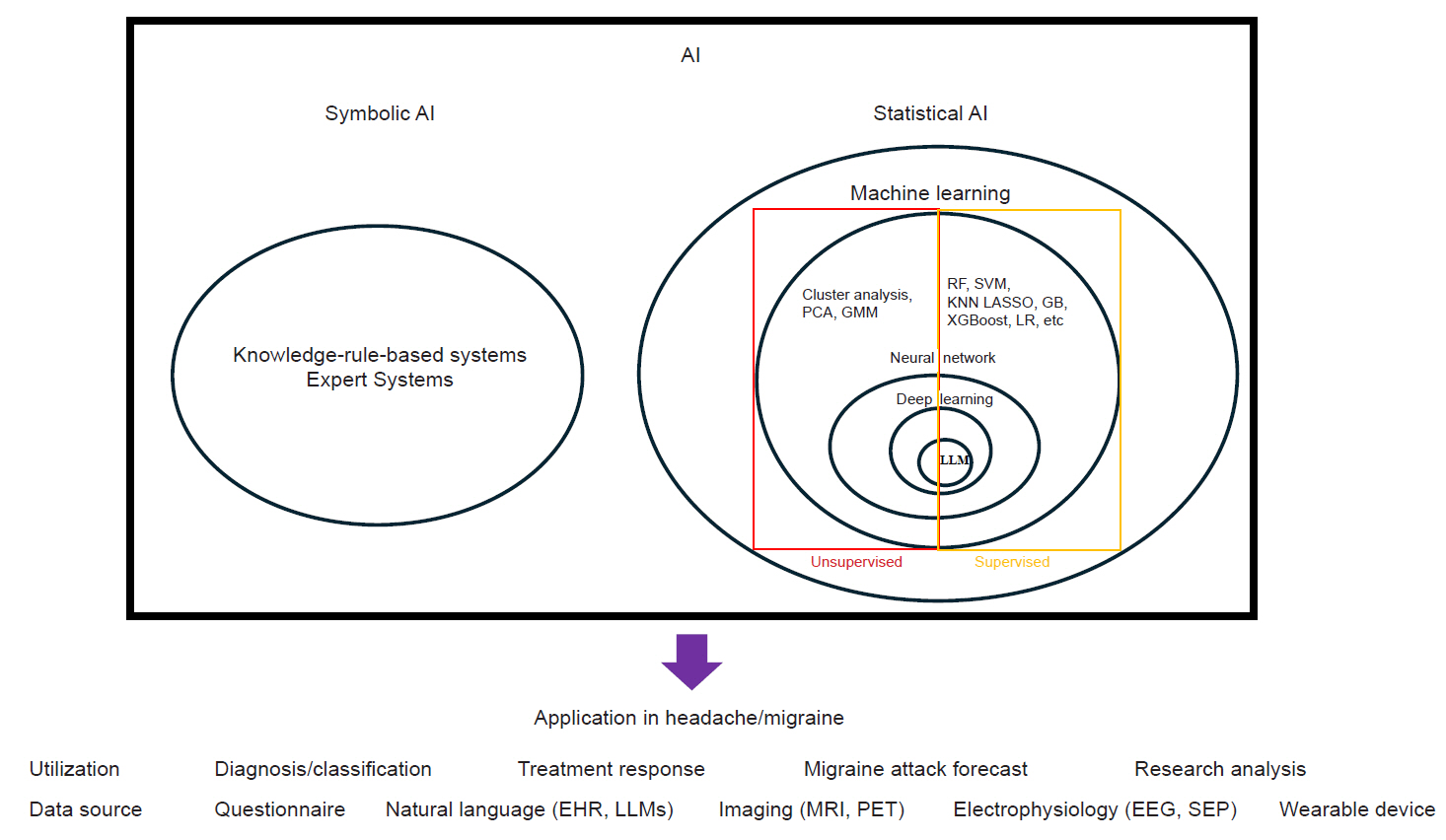
Schematic diagram of AI and its applications in the headache field AI can be divided into symbolic and statistical methods. Machine learning, neural networks, deep learning, and LLMs are examples of statistical methods. These methods can also be categorized as unsupervised or supervised based on their use of labeled data. The applications of AI in headache and migraine can be analyzed in terms of its utilization and the data source.
AI, artificial intelligence; PCA, principal component analysis; GMM, Gaussian mixture models; RF, random forest; SVM, support vector machine; KNN, K-nearest neighbor; LASSO, least absolute shrinkage and selection operator; GB, gradient boosting; XGBoost, extreme gradient boosting; LR, logistic regression; LLM, large language model; EHR, electronic health records; MRI, magnetic resonance imaging; PET, positron emission tomography; EEG, electroencephalography; SEP, somatosensory evoked potentials.
Examples of symbolic AI include Deep Blue for chess gameplay and MYCIN in the medical field, a computer-based consultation system designed to assist physicians in the diagnosis and therapy selection for patients with bacterial infections.4
The evolution of computer systems has driven the rapid advancement of AI technologies, particularly in the area of statistical AI. Statistical methods can be divided into ‘supervised’ and ‘unsupervised’ learning, based on whether they have answers, known as ‘labels.’ Machine learning (ML) is a type of statistical AI that involves algorithms for data-driven pattern analysis, decision-making, and prediction. Among ML algorithms, neural networks are models inspired by the human neural network. Among artificial neural networks (ANN), convolutional neural networks (CNN) are better suited for image analysis, while recurrent neural networks and long short-term memory networks are more appropriate for linear and wavelet data. DL refers to neural network algorithms with multiple, deep layers. Numerous DL architectures are available, each proven effective for specific type of data.
Utilization of AI in headache medicine can be categorized into several key areas: diagnosis or classification of headache disorders, assessment of treatment response, forecasting of migraine attacks, and as a tool for analysis during research. Regarding data sources and methods, AI applications utilize a range of inputs including questionnaires, language data (e.g., generative language models or electronic health records [EHR]), medical devices or tools such as magnetic resonance imaging (MRI), results from electrophysiology studies (e.g., electroencephalography [EEG], somatic evoked potential [SEP]), and wearable devices, either individually or in combination. This review aims to outline the current use and role of AI in the field of headache disorders, with a focus on migraine, and to discuss future perspectives.
METHODS
1. Search strategies
Although this is not a systematic review, the search process was conducted following the Preferred Reporting Items for Systematic Reviews and Meta-Analyses (PRISMA) guidelines.5 A literature search was performed in PubMed using the following terms:
((migraine*) AND ((artificial*) OR (artificial intelligence*) OR (AI*) OR (deep learning*) OR (machine learning*) OR (artificial intelligence [MeSH Terms]) OR (AI [MeSH Terms]) OR (deep learning [MeSH Terms]) OR (machine learning [MeSH Terms])))
The search was restricted to literature published between 1 January 2000 and 31 July 2024. Only abstracts in the English language were included for review.
2. Inclusion and exclusion criteria
In reviewing abstracts, only studies that explicitly included AI, ML, or DL methods in their analytical processes were considered for inclusion. Studies where the authors used ML or DL methods but did not specify this in the abstract were excluded. Semi-automated approaches that involved computational methods alongside expert-suggested algorithms were included if they were specified as AI-based methods or if they were well-organized for comparative review. Medical tools, including imaging techniques such as MRI and positron emission tomography (PET), electrophysiology methods such as EEG and SEP, magnetoencephalography (MEG), and other devices such as wearable technologies, were included if the analytical methods utilized AI techniques. Review articles, editorials, opinions, and viewpoints were considered for snowballing purposes but were generally excluded from the systematic review. Additionally, studies for which the full text was unavailable or not published in English were excluded. Manuscripts were further excluded if they employed inappropriate methodologies, such as not applying the International Classification of Headache Disorders, 3rd edition (ICHD-3) criteria, or if they involved improper headache diagnosis or did not specify headache participants.
RESULTS
1. Search results and article inclusion/exclusion
Of the 398 articles identified, one was a duplicate and 317 were excluded based on abstract review. Of the remaining 80 articles, six were review articles, four were editorials or opinion pieces, two did not utilize AI methodology, one was not related to the headache field, seven did not adhere to ICHD-3 criteria or did not specify headache diagnosis methods, and four had full texts that were unavailable. An additional five articles were identified through the snowball technique. In total, 61 articles published between 2002 and 2024 were included in the review, with the majority published since 2020. The PRISMA flow chart outlining the selection process is shown in Figure 2. The summaries of the included studies are demonstrated in Table 1.
ARTIFICIAL INTELLIGENCE APPLICATIONS IN THE DIAGNOSIS OF HEADACHE DISORDERS
1. Questionnaire/survey
Traditionally, questionnaires have been valuable tools in aiding the diagnosis of headache disorders, given that such diagnoses are typically based on clinical profiles. Furthermore, previously collected data from these questionnaires facilitates the swift and effective application of AI technology.
The number of items in the questionnaires varied from 17–226-12 to 75.13 While the details differed, all questionnaires included demographic data (age, sex), headache characteristics, duration, frequency, and accompanying symptoms.
The number of participants and the number of classifying groups varied across studies. Liu et al.6 distinguished between 84 migraine and 89 tension-type headache (TTH) participants using a 19-item questionnaire. Simić et al.7 utilized a 20-item questionnaire to classify 1,022 subjects, identifying 169 with migraine, 224 with TTH, and 186 with other headache types. Kwon et al.13 employed a 75-item questionnaire from a headache center to classify 2,162 individuals with headache disorders, including migraine, TTH, trigeminal autonomic cephalalgias (TAC), epicranial headaches, and thunderclap headaches.
Most studies utilized supervised ML methods, including decision trees (DTs), random forests (RFs), gradient boosting (GB), logistic regression (LR), and support vector machines (SVMs). The performances were presented with sensitivity, specificity, accuracy, area under the receiver operating characteristic curve (AUROC), and F1 score. The F1 score is the harmonic mean of precision and recall (
Kwon et al.13 used a stacked classifier model with four layers of eXtreme Gradient Boosting (XGBoost) classifiers, each layer classifying migraine, TTH, TAC, epicranial headaches, and thunderclap headaches. Different features were selected from the self-reported data at each layer using the least absolute shrinkage and selection operator (LASSO). The model achieved an accuracy of 81% for the test set. The sensitivity and specificity for migraine, TTH, TAC, epicranial headache, and thunderclap headache were 88% and 95%, 69% and 55%, 65% and 46%, 53% and 48%, and 51% and 51%, respectively.13
In contrast, Simić et al.7 proposed a hybrid system incorporating the Calinski-Harabasz index, Analytical Hierarchy Process, and Weighted Fuzzy C-means Clustering algorithm, an unsupervised ML method. The accuracy rates were 67% for migraine, 74% for TTH, and 86% for other primary headaches, with corresponding F1 scores of 75%, 74%, and 75%, respectively.7
The Japanese research group, led by Katsuki, Yamamoto, Sasaki, and Okada, along with other co-authors, has published multiple articles utilizing questionnaires and AI methods. In their first study, published in 2020, they used a combination of questionnaires, unstructured descriptions, and DL methods to classify primary headaches among 848 participants, with 46% diagnosed with migraine, 47% with TTH, and 5% with TAC.14 Natural language processing (NLP) was employed using the commercial DL framework, Prediction One, and an ANN model was applied. The model achieved an accuracy of 0.7759, a mean precision of 0.8537, a mean recall of 0.6086, and a mean F1 score of 0.6353.
In subsequent studies, the same group used a 17- or 22-item questionnaire along with multiple AI methods to classify five to six different outcomes: migraine and medication-overuse headache (MOH) separately or together as migraine/MOH, TTH, TACs, other primary headaches, and other headaches.10 Among the 6,058 participants, there were 4,829 cases of migraine, 834 cases of TTH, 78 cases of TACs, 38 cases of other primary headache disorders, and 279 cases of other headaches. The GB classifier yielded the highest c-statistic of 0.88. The c-statistic, equivalent to the AUROC, measures a classification model’s ability to discriminate between classes, with higher values indicating better performance. The model’s accuracy, sensitivity, specificity, precision, and F1-score were 93.7%, 84.2%, 84.2%, 96.1%, and 84.2%, respectively.
The AI model’s performance was compared with that of non-headache specialists, and its usefulness in aiding headache diagnosis was evaluated using data from a study of 4,000 patients.8 The light GB machine classifier achieved the highest c-statistic of 0.9203. The diagnostic accuracy of five non-headache specialists was then compared to that of the AI model using a sample of 50 patients. Without the AI model, the non-specialists’ overall diagnostic accuracy was 46%, with a kappa value of 0.212. With the aid of the AI model, their accuracy and kappa value improved significantly to 83.2% and 0.678, respectively. External validation of the AI model’s diagnostic performance using a sample of 59 participants demonstrated an overall accuracy of 94.92% and a kappa value of 0.65 (95% confidence interval [95% CI], 0.21–1.00) when compared to the ground truth. The sensitivity, specificity, precision, and F1-score for diagnosing migraines were 98.21%, 66.67%, 98.21%, and 98.21%, respectively.11
The application of the system in pediatric and adolescent populations was also validated. Sasaki et al.12 used multiple AI models to diagnose 909 participants aged 6 to 17 years, including 234 individuals with migraine. For the test dataset, the model achieved an accuracy of 94.5%, sensitivity of 88.7%, specificity of 96.5%, precision of 90.0%, and an F1-score of 89.4%.
However, non-AI methods and rule-based decision systems have also demonstrated impressive results. For example, a web-based headache diagnosis questionnaire validated by telephone interviews showed a sensitivity of 92.6%, a specificity of 94.8%, and a kappa coefficient of 0.875 for diagnosing migraine among 256 participants. For the diagnosis of TTH and probable migraine (PM), the sensitivity, specificity, and kappa coefficients were 78.4%, 98.4%, and 0.809, and 85%, 92.9%, and 0.757, respectively.15
Computerized systems based on expert opinions have also proven effective. In 2008, Maizels and Wolfe16 developed a Computerized Headache Assessment Tool (CHAT) using web-based questionnaires with branching questions based on headache frequency, duration, and ICHD criteria. Among 135 participants who completed CHAT and 117 who completed a diagnostic interview, CHAT correctly identified 35/35 cases (100%) of episodic migraine (EM), 42/49 cases (85.7%) of transformed migraine, 11/11 cases of chronic TTH, 2/2 cases of episodic TTH, and 1/1 case of episodic cluster headache (CH). It also identified medication overuse in 43/52 cases (82.7%), with the most common misdiagnosis being transformed migraine or new daily persistent headache.
In another study by Cowan et al.17, the concordance between a self-administered, computer-based diagnostic engine (CDE) and a semi-structured interview conducted by a headache specialist was assessed. The CDE, developed by the authors using a detailed DT, was completed by 212 participants, who also underwent an interview. For diagnosing migraine and PM, the CDE demonstrated a sensitivity of 90.1% and a specificity of 95.8%, with a concordance rate with SSI of κ=0.83 (95% CI, 0.75–0.91).
These expert-based systems, built on transparent decision-making processes using ICHD-3 criteria, exhibit high sensitivity and specificity. In contrast, AI operates as a “black-box” system, where the decision-making process is not easily interpretable. While AI models may demonstrate high accuracy, careful interpretation according to current knowledge is necessary, and biases of the data may result in subpar prediction results.18 Questions remain as to whether current AI offers real advantages beyond being novel and innovative. The challenge remains validating AI models and ensuring their effective application in real-world settings.
2. Natural language
Natural language as a data source in headache research holds significant potential, especially in aiding practitioners and saving time. Many patient interviews are naturally conducted in unstructured language, which doctors traditionally summarize and interpret to make a diagnosis. While structured questionnaires have been used to standardize this natural language, the raw language itself may contain even more valuable information. In this context, natural language includes any unstructured text, such as EHRs and generative large language models (LLMs). However, studies utilizing generative LLMs have predominantly focused on assessing treatment response rather than diagnosis and classification. Three studies were identified in the area of diagnosis and classification, with one integrating questionnaire data and natural language, as previously discussed in the questionnaire section.
Riskin et al.19 used US claims and EHR data from 2010 to 2012 to compare the efficacy of migraine identification. They defined “Traditional Real-World Evidence (RWE)” as the use of insurance claims or structured EHR data, while “Advanced RWE” was defined as the use of unstructured EHRs. Although the exact AI-based technology was not specified, an ML algorithm was employed. Based on manual annotation by seven annotators, 2,642 migraine and 6,530 headache-related concepts were identified, and their recall rates were compared. “Traditional RWE” achieved recall rates of 66.6% and 29.6%, while “Advanced RWE” recalled 96.8% and 92.9%, respectively. The superior performance of “Advanced RWE” was consistent across the identification of six migraine-associated symptoms, with F1 scores ranging from 80.7% to 95.6%.
Vandenbussche et al.20 conducted a web-based survey in which 81 migraine and 40 CH patients were asked to describe their headache disorders in detail. NLP was applied to analyze the narrative self-reports, focusing on lexical, semantic, and thematic properties. Lexicon-based sentiment analysis of attack descriptions revealed predominantly negative sentiments. For the classification of migraine and CH using features from the attack descriptions, LR and SVM algorithms demonstrated the best performance, with F1 scores ranging from 0.6 to 0.8. There was a significant difference between Dutch-speaking migraine and CH patients in how they described their disorder. Migraine patients used the Dutch word for “headache” more often, while CH patients more frequently used the word “pain.”
3. Imaging
Numerous studies have employed brain imaging techniques, such as MRI, functional MRI (fMRI), and PET, analyzed with ML and DL methods to differentiate and classify headache disorders, particularly migraine.
Mitrović et al.21 analyzed brain MRI data from a cohort including healthy controls (HCs) and patients with migraine with aura (MwA). Cortical thickness, surface area, and volume were compared using various ML methods.21 The best classification results were obtained with linear discriminant analysis (LDA), achieving 97% accuracy for MwA. Left temporal pole, right lingual gyrus, and left pars opercularis thickness were notable distinguishing features. Further research used the average Migraine Aura Complexity Score (MACS) from multiple MwA attacks and evaluated its correlation with 340 MRI features.22 Applying ML methods including SVM, a high coefficient of determination (0.89) was achieved, with 26 significant features including left parahippocampal mean Gaussian curvature, left transverse temporal mean Gaussian curvature, left transverse temporal thickness, and left pars opercularis thickness (p<0.01) strongly correlating with average MACS (p<0.05).
Chong et al.23 combined questionnaire data with T1-weighted MRI and diffusion tensor imaging, to distinguish between migraine and persistent post-traumatic headache (PTH) attributed to mild traumatic brain injury. A logistic classifier achieved an overall accuracy of 78%, with 97.1% accuracy for migraine and 64.6% for PTH. Critical features contributing to accuracy included responses related to anxiety on sports concussion Assessment Tool and decision-making difficulty on Beck Depression Inventory-13, as well as cortical brain regions such as the bilateral superior temporal gyrus, inferior parietal lobe, posterior cingulate cortex, and fiber tracts like the right anterior thalamic radiations and right superior longitudinal fasciculus. Additional study utilized clinical data, along with MRI measures of brain structure and functional connectivity.24 A classifier using ridge LR on principal components achieved an average accuracy of 72% when using functional connectivity data, and 63.4% without it. In addition, a DL method was developed using a 3D ResNet-18 classifier to automatically identify features that differentiate MRIs of 95 migraine patients, 48 with acute PTH, 49 with persistent PTH, and 532 HCs. The 3D ResNet-18 classifier, an 18-layer CNN based DL architecture for image analysis, adapted for 3D convolutions, achieved an accuracy of 75%, a sensitivity of 66.7%, and a specificity of 83.3% in distinguishing migraine from HCs. The most significant biomarkers identified by the migraine classifier included the caudate, caudal anterior cingulate, superior frontal gyrus, thalamus, and ventral diencephalon.25
Resting-state fMRI has been frequently analyzed in migraine research, utilizing various ML and DL techniques for feature extraction and classification. Several studies compared migraineurs and HCs.
Tu et al.26 examined 70 migraine without aura (MwoA) patients and 46 matched HCs, identifying abnormal functional connectivity within the visual network (VN), default mode network (DMN), sensorimotor network (SMN), and fronto-parietal networks that distinguished migraineurs from HCs using an SVM model with 93% sensitivity and 89% specificity. The model was validated on an independent cohort of 19 MwoA patients and 19 additional controls, achieving 84% sensitivity and specificity. To verify specificity, the model was tested on 18 MwoA patients and 76 non-migraine pain patients (with chronic lower back pain and fibromyalgia), demonstrating 78% sensitivity and 76% specificity for distinguishing migraineurs from non-migraineurs.
Nie et al.27 applied both unsupervised and supervised ML techniques. Using an automatic segmentation algorithm, K-means clustering combined with hierarchical clustering identified 17 dynamic functional connectome patterns (DFCPs).27 SVM was used to select optimal features from static functional connectivity strength and DFCP features and to classify migraine patients and HCs.28
Chong et al.29 used diagonal quadratic discriminant analysis (QDA), an ML algorithm to analyze functional connections from 33 seeded pain-related regions of 58 migraine patients and 50 HCs. Notably, those with an disease duration of more than 14 years were classified more accurately (96.7% vs. 82.1%).
MwA was also examined in several studies. Fernandes et al.30 used Gaussian Process Classifier to differentiate between ictal and interictal periods in two patients with MwA.
Yang et al.31 analyzed the amplitude of low-frequency fluctuations, regional homogeneity, and regional functional correlation strength to distinguish 21 patients with MwoA, 15 with MwA, and 28 HCs. SVM classifier achieved an accuracy of 83.67%, whereas a CNN approach based on the Inception module improved accuracy to 86.18%.
4. Electrophysiology and magnetoencephalography
Wavelet data from electrophysiology studies, including EEG and SEP, have also been utilized for the diagnosis and classification of migraine. Analyzing these data often requires transformations, such as Fourier transformation, to process the complex signals. MEG has also been employed in the analysis of headache disorders and, in this review, is included in this section due to its time-dependent data acquisition characteristics. Studies utilizing EEG and MEG signals have been conducted to differentiate migraine from other conditions.
Hsiao et al.32 conducted multiple studies utilizing MEG. In 2022, resting-state MEG data from 70 HCs, 100 chronic migraine (CM) patients, 35 EM patients, and 35 FM patients were analyzed to calculate source-based oscillatory connectivity in relevant cortical regions.32 Using a SVM classifier, a model was developed to identify CM. The salience, SMN, and parts of the DMNs were key features differentiating CM from HCs, with classification performance showing an accuracy of ≥86.8% and an area under the curve (AUC) of ≥ 0.9. When comparing CM to EM, the model achieved an accuracy of 94.5% and an AUC of 0.96, and for CM versus FM, an accuracy of 89.1% and an AUC of 0.91. In 2023, resting-state MEG data of 70 HCs, 100 CM, 40 CM with FM, 35 FM, 30 chronic TTH, and 75 EM were analyzed.33 Features were extracted and classified using ML algorithms including DT, discriminant analysis, naïve Bayes classifiers, SVM, and K-nearest neighbor (KNN). The best classification model distinguished CM from HCs with an accuracy of over 92.6% and an AUC of over 0.93. When validating CM classification against other groups, accuracy exceeded 75.7%, with an AUC greater than 0.8.
Although EEG is not routinely recommended in headache practice, its application in headache research has persisted.34 EEG signals have been utilized to classify HCs, migraine patients, CM patients,35,36 and to differentiate between MwA and MwoA.37 EEG signals were recorded during resting state, visual or auditory stimulation tasks, or non-painful, painful, and repetitive painful electrical stimulation. Various signal processing techniques were applied, such as the tunable Q-factor wavelet transform method to decompose EEG signals into sub-bands38 and segmentation of a 3-minute EEG into 120 1-second segments, generating 325 functional connectivity values between electrode pairs.37 Most studies employed ML models. However, in one study, EEG signals were transformed into scalogram-spectrogram images and classified using CNN architectures, including AlexNet, ResNet50, and SqueezeNet.36
Akben et al.39 in 2012 compared different flash stimulation frequencies (2 Hz, 4 Hz, and 6 Hz) and durations (2 seconds, 4 seconds, 6 seconds, and 10 seconds) to determine the most effective conditions for detecting migraine. EEG was recorded during flash stimulation in 15 migraine patients and 15 HCs. The power spectral density estimate was computed, and a multilayer perceptron (MLP) neural network was used for classification. The study found that a 4 Hz flash stimulation frequency and an 8-second duration were most effective in detecting migraine, particularly at the beta band of the T5-T3 channel.
In another study by Cao et al.40, a wearable, wireless EEG device (Mindo-4S) was used to record EEG signals from the prefrontal (Fpz) and occipital (O1, Oz, O2) regions to differentiate 40 MwoA patients from 40 HCs. EEGs from interictal, pre-ictal, ictal, and post-ictal phases were processed, and a binary classification model was developed using LDA, KNN, MLP, Bayesian classifier, and SVM. The SVM demonstrated the highest accuracy (76%±4%) for classifying interictal and pre-ictal phases using prefrontal EEG complexity.
Chiang et al.41 analyzed the electrocardiogram (ECG) data of 17,840 participants with MwA and 22,162 participants with MwoA, excluding those with a history of atrial fibrillation (AF). The team employed an AI-ECG algorithm, developed using a CNN-based approach, to calculate the probability of concurrent paroxysmal or impending AF in ECGs showing normal sinus rhythm. The AF prediction model output was significantly higher in the MwA group compared to the MwoA group (mean [standard deviation], 7.3% [15.0%] vs. 5.6% [12.4%]; mean difference [95% CI], 1.7% [1.5%–2.0%]; p<0.001). These differences remained significant even after adjusting for vascular comorbidities, suggesting a higher probability of concurrent paroxysmal or impending AF in individuals with MwA compared to those with MwoA.
Although not as extensively researched, SEP have also been investigated in the context of migraine. Zhu et al.42 utilized SEP data to differentiate between 42 migraine patients (29 in the interictal phase and 13 in the ictal phase) and 15 HCs. The right median nerve SEPs were recorded, and features in both the time and frequency domains were selected through a feature selection method. The data were then classified using various ML algorithms, including RF, XGBoost trees, SVM, KNN, MLP, LDA, and LR. The classification accuracies for distinguishing HCs, ictal, and interictal phases ranged from 51.2% to 72.4%. After model and feature selection, the accuracy improved to 89.7% for HC-ictal, 88.7% for HC-interictal, 80.2% for ictal-interictal, and 73.3% for HC-ictal-interictal classification. Interestingly, a tested CNN-based model showed lower performance compared to the ML-based models.
5. Wearables and other devices
De Brouwer et al.43 utilized the Empatica E4 wearable device (Empatica Inc., Cambridge, MA, USA) along with a custom-made application to maintain a diary of headache-specific data. The device employed data-driven ML algorithms to detect activity, stress, and sleep events. Individual headache attacks were classified based on a knowledge-based classification system, focusing on migraine, CH, and TTH. A total of 133 headache attacks from 14 migraine and four CH patients were analyzed. The strict application of ICHD-3 criteria resulted in the classification of eight out of 98 MwoA attacks and 0 out of 35 CH attacks. However, an adapted version of the criteria, which modified the headache duration for treated and terminated episodes, improved classification to 28 out of 98 MwoA attacks and 17 out of 35 CH attacks. The device also collected data on activities and stress events, which were confirmed in 46% and 59% of cases, respectively, indicating the potential link between headache and physiological data, although further improvement is warranted.
Functional near-infrared spectroscopy was employed to measure changes in hemoglobin levels in the prefrontal cortex during a mental arithmetic task, with the data used to classify 13 HCs, nine CM patients, and 12 MOH patients.44 ML techniques, including LDA and QDA, were applied in both direct and stepwise classifications. The resulting model achieved a sensitivity of 100% and a specificity of 75% in classifying CM patients.
The statistical application of AI is particularly well-suited for use in classification tasks, especially when applied to data sources such as brain imaging, electrophysiology, wearable devices, or other measurable inputs. These data sources provide numerous inputs, and the diagnosis of headache disorders offers clearly defined target labels, facilitating the use of AI in generating accurate classifications. As demonstrated in the studies presented, these methods often yield favorable accuracies and show significant potential. However, the application of these AI methods in real-world clinical settings remains uncertain. A meta-analysis on the real-world accuracy of wearable activity trackers for detecting COVID-19, AF, and falls reported sensitivities of 79.5%, 94.2%, and 81.9%, and specificities of 76.8%, 95.3%, and 62.5%, respectively.45 Notably, the highest accuracy was observed in detecting AF, which is primarily diagnosed using wavelet-transformed data from ECG signals. In contrast, the gold standard for diagnosing headache disorders is patient interviews, and interpreting headache diagnoses classified by complex wavelet data presents significant challenges. Additionally, randomized controlled studies are limited in demonstrating the benefits of AI or comparing with gold standard methods.18 Also most studies, except for the ECG study by Chiang et al.46, involved a small number of participants, raising concerns about the generalizability of these AI applications to broader populations.
ARTIFICIAL INTELLIGENCE APPLICATIONS IN THE ASSESSMENT OF TREATMENT EFFICACY AND RESPONSE IN HEADACHE DISORDERS
Assessing treatment response is a crucial aspect of clinical practice. Identifying responders and non-responders helps avoid ineffective therapies and minimize adverse effects, which is the core principle of precision medicine. This is particularly important when treating patients with headache disorders, especially CM, using costly therapies such as OnabotulinumtoxinA and anti-calcitonin gene-related peptide monoclonal antibodies (anti-CGRP mAb), where non-responders can have significant implications. AI methods have been increasingly utilized to assess or predict the need for treatment, evaluate treatment response, and identify potential good responders.
1. Questionnaire/survey
Ashina et al.47 conducted a web-based survey involving 31,529 out of 61,826 individuals (51.0%) who had sought medical care for migraine in the previous 12 months. Using ML techniques, including RF and LASSO, the study identified 13 sociodemographic and clinical factors most strongly associated with seeking medical care for migraine. Among these, higher interictal burden, disability, and allodynia were particularly significant factors.
2. Natural language
NLP of EHRs, generative LLMs have been utilized to assess treatment response, evaluate current treatment status, and analyze patient feedback.
Hindiyeh et al.48 constructed a migraine outcome model based on headache severity (mild, moderate, severe), headache descriptors (pulsating, debilitating, stabbing), headache progression, and associated symptoms (nausea, vomiting, photophobia, and phonophobia). Each data element was weighted to define a 10-point scale. EHR data from 2018 to 2020 were reviewed, and trained annotators assigned scores. The accuracy of “traditional approaches” and “advanced approaches” was compared. From 2,006 encounters, the average F1 score for automated extraction was 92.0% for AI applied to unstructured data (advanced approach).
Guo et al.49 developed a platform-independent text classification system to automatically detect and analyze self-reported migraine-related posts. Texts from Twitter and Reddit were manually labeled, and six transformer-based models were used to classify posts as positive if at least one sentence within the post was identified as self-reporting. The best system achieved an F1 score of 0.9 on Twitter and 0.93 on Reddit, demonstrating minimal bias. Treatment-related information and associated sentiments were also analyzed. This study suggests the potential for analyzing treatment response based on real-time, real-world self-reports, outside of traditional hospital settings or headache diaries, which could reduce recall bias.
Chiang et al.50 performed a retrospective cross-sectional study from two tertiary headache referral centers. A total of 1,915 neurology consultation notes written by 15 specialized clinicians between 2012 and 2022 were extracted. Four NLP frameworks were applied to generate answers and extract headache frequency. Among these, the generative pre-trained transformer 2 (GPT-2) generative model showed the best performance, with an accuracy of 0.92 (95% CI, 0.91–0.93) and an R2 score of 0.89 (95% CI, 0.87–0.90). All GPT-2–based models outperformed the ClinicalBERT (Bidirectional Encoder Representation from Transformers) model in terms of exact matching accuracy.
Li et al.51 provided 30 migraine-related queries, including evaluation, definition, testing, diagnosis, treatment, follow-up, prognosis, and special population considerations, to five LLMs (ChatGPT-3.5, ChatGPT-4.0, Google Bard, Meta Llama2, and Anthropic Claude2). The answers were randomly ordered and rated by neurologists.51 Although the difference in performance was not statistically significant, ChatGPT-4.0 received the highest accuracy ratings, whereas Google Bard had a relatively higher proportion of ‘poor’ ratings. Notably, there were erroneous recommendations, such as proposing hemicraniectomy for persistent and severe migraine by ChatGPT-3.5.
This study highlights the need for caution among clinicians, researchers, and potential patients when using LLMs for medical purposes. These erroneous recommendations are not just incorrect; they have the potential to cause patient harm. Therefore, the use of LLMs must be managed with caution and public awareness, and further research is warranted.
Another significant caution regarding the use of LLMs for medical advice arises from a study by Moskatel and Zhang.52 They queried ChatGPT-3.5 on the efficacy of 47 medications for the prevention of migraine and evaluated its responses and citations. The assessments of 33 medications were found to be unreliable, with 66% (76/115) of the citations being hallucinations and 5% (6/115) being erroneous.
3. Clinical dataset
Lu et al.53 evaluated 610 migraine patients, including 326 who responded to non-steroidal anti-inflammatory drugs (NSAIDs) and those who did not. They extracted potential predictors among demographic and clinical features using multivariable LR analysis.53 The SVM, DT, and MLP algorithms were used to predict NSAID responsiveness, with the AUC for the test cohort ranging from 0.712 to 0.744 across the three ML methods. Significant predictors identified included disease duration, headache intensity, frequency, anxiety, depression, and sleep disorders.
Martinelli et al.54 attempted to predict treatment response to OnabotulinumtoxinA in patients with CM and high-frequency episodic migraine. Among the 212 enrolled patients, 35 were classified as excellent responders and 38 as non-responders. The Relif Family feature selection algorithm was used to select demographic and clinical data, which were then analyzed using various ML methods. Although ML methods failed to distinguish good responders from non-responders overall, the RF algorithm in the high-frequency EM group achieved a high classification accuracy of 85.71%. Key predictors of response in the high-frequency EM group included age at migraine onset, opioid use, anxiety subscore on the Hospital Anxiety and Depression Scale, and Migraine Disability Assessment (MIDAS) score.
Gonzalez-Martinez et al.55’s team utilized prospectively collected multicenter dataset of 712 migraine patients receiving anti-CGRP mAb therapies to predict treatment response. The study population was predominantly female (93%), with 84% having CM. A RF-based approach was employed, with hyperparameters selected using a Bayesian search optimization method. Prediction models at 6, 9, and 12 months utilized variables such as headache days per month at each time point and their reduction, migraine days per month at baseline and 3 months, and headache impact test (HIT-6) scores. The F1 scores of the models ranged from 0.70 to 0.97, with AUROC values between 0.87 and 0.98. A calculator tool was subsequently developed and made available online (https://portal.brainguard.life/tools/cgrp.php).
Stubberud et al.56 utilized clinical data from a retrospective cohort of 1,446 CM patients to estimate individual treatment effects across 10 classes of preventive therapies, including OnabotulinumtoxinA, flunarizine, candesartan, serotonin-noradrenaline reuptake inhibitors, topiramate, tricyclic antidepressants, acupuncture, valproate, beta blockers, and serotonergic agents. The analysis was performed using a causal multitask Gaussian process model. Data were collected through automated extraction using NLP of Microsoft Word template-based clinical records, achieving an accuracy of 90.73% compared to manual extraction. Individual treatment effects were then used to rank the preventive therapies for machine-guided prescription. The machine prescription policy was estimated to reduce time-to-response by 35% (3.750 months; 95% CI, 3.507–3.993; p<0.0001) compared with expert guidelines, with no substantive increase in expense per patient.
Ferroni et al.57’s research utilized a dataset of 777 migraine patients with 21% (162) of whom reported MO lasting for at least 2 years, to predict the risk of developing MO. The team developed a customized ML-based decision support system combining SVM and Random Optimization (RO-MO), which was compared to a baseline SVM model. The final RO-MO decision support system, incorporating the top four models, achieved a c-statistic of 0.83, with sensitivity and specificity of 0.69 and 0.87, respectively, and an accuracy of 0.87. LR analysis confirmed the system’s effectiveness in predicting MO, with odds ratios of 5.7 and 21.0 for patients classified as probably (three predictors positive) and definitely at risk of MO (four predictors positive), respectively.
Ciancarelli et al.58 used ANN to predict the effect of EMG-biofeedback treatment in 20 CM patients. The ANN predicted post-treatment MIDAS scores with 75% accuracy. A significant correlation between NOx (nitrite and nitrate) levels and MIDAS (R=−0.675, p=0.011) suggested that higher nitric oxide levels pre-treatment were associated with lower post-treatment MIDAS scores, particularly when peroxide levels are within a specific range (116–205 U/mL).
4. Imaging
Wei et al.59 evaluated 111 migraine patients, of whom 62 were responders to NSAIDs and 49 were non-responders. Their 3D-T1 weighted images were analyzed using DL with the ResNet-18 model demonstrated the best accuracy of 0.78. In a subsequent study, the static functional connectivity was compared among 35 NSAID-responsive episodic MwoA patients, 35 NSAID-non-responsive MwoA patients, and 33 HCs. Clinical characteristics and functional network connectivity features were applied to a SVM model to classify NSAID responsiveness, yielding a sensitivity of 0.88, specificity of 0.89, and an AUROC of 0.93. NSAID-responsive patients exhibited reduced connectivity between the DMN and VN, as well as between the SMN and VN, while showing enhanced VN-auditory network connections.
In a follow-up study, the team compared 59 NSAID responders with 59 non-responders among migraine patients, using propensity score matching.60 Multimodal MRI was employed to extract percentage amplitude oscillations and gray matter volume from six brain areas, with multiple ML models applied. The RF model, which had the lowest predictive residuals, was selected. The model metrics in the training and testing groups were as follows: AUROC 0.982/0.711, sensitivity 0.976/0.667, and F1 score 0.930/0.649. The choice of AI algorithm is noteworthy. ResNet-18, a CNN based DL architecture, is advantageous for direct image analysis. When features extracted from MRI were used, ML methods were applied. Marino et al.61 utilized Compressive Big Data Analytics (CBDA), a semi-supervised ML technique, to identify predictive migraine biomarkers at the molecular level using a PET dataset from 38 migraine patients and 23 HCs. The CBDA method classified migraineurs from HCs with accuracy, sensitivity, and specificity above 90% for both whole-brain and region-of-interest analyses. The putamen was identified as the most predictive region for migraine, particularly regarding μ-opioid and D2/D3 dopamine receptors.
Tso et al.62 predicted verapamil responsiveness in 708 CH and probable CH patients, comprising 317 episodic and 391 chronic cases, using 72 clinical features from 410 patients and imaging data from 194 patients. Non-linear dimensionality reduction techniques, including principal component analysis and t-distributed stochastic neighbor embedding, were applied to the clinical data, identifying two large clusters. KNN was then used to define these clusters. The voxel-based morphometry analysis revealed a gray matter cluster in lobule VI of the cerebellum (–4, –66, –20) that exhibited increased gray matter concentration in verapamil non-responders compared with responders (p=0.008). The XGBoost-implemented GB DT was used to predict verapamil response, achieving AUROC of 0.689 on cross-validation (95% CI, 0.651–0.710) and 0.621 on held-out data.
While there are still relatively few studies and the results have not yet been particularly compelling, the potential for utilizing AI in this area has been demonstrated. Further research and development are needed to refine these methods and make them more accessible for clinical application in the future.
ARTIFICIAL INTELLIGENCE APPLICATIONS IN MIGRAINE ATTACK PREDICTION
1. Forecasting migraine attack
Migraine sufferers often have a strong desire to predict both the onset and intensity of a migraine attack. Despite knowing that acute-phase migraine medication should be taken immediately when a headache begins (as reported by 184 out of 207 participants), many delay treatment. This hesitation is largely due to the desire to confirm whether the headache is indeed a migraine (68.7%) and to reserve medication for cases that develop into severe migraine attacks (46.2%).63 The application of AI holds great potential in forecasting migraine attacks, given its strength in classification and prediction.
In a study by Stubberud et al.64, 18 migraine patients were prospectively included, completing 388 headache diary entries and self-administering app-based biofeedback sessions that wirelessly measured heart rate, peripheral skin temperature, and muscle tension. The primary outcome was the presence or absence of any headache on the day following a completed headache diary entry and biofeedback session. The RF model was the top-performing model in the out-of-sample test set, achieving an AUROC of 0.62, with accuracy, sensitivity, and specificity of 0.56, 0.0, and 1.0, respectively. A GB classifier showed similar results. Using SHapley Additive exPlanations, the most important features for predicting the next day’s headache were identified as premonitory symptoms (craving, swelling, and feeling cold), the amount of sleep, the presence and intensity of headache, the impact of the headache on daily functioning, the length of the biofeedback session, and mean heart rate.
Siirtola et al.65 utilized wearable sensors from the wrist-worn Empatica E4 device, along with sleep data, to predict migraine attacks. Data from seven participants, including headache diaries and sleep metrics, were used. The wearable device collected data from a 3D accelerometer, thermometer, electrodermal activity sensor (galvanic skin response), and photoplethysmography sensor (measuring blood volume, heart rate, and heart rate variability). Features were derived by comparing nights before a migraine attack to nights without an attack, and nights before a day without a migraine were also compared with each other. QDA and LDA were used as classifiers, with QDA producing better results than LDA. The personal model outperformed the balanced user-independent model, with accuracy for detecting attacks one night prior exceeding 82% in five individuals, while accuracy varied significantly, ranging from 60.4% to 69.6% in the other two individuals.
Katsuki et al.9 utilized a smartphone application to collect hourly headache occurrences from 4,375 migraine sufferers, integrating this data with local weather information. The variables were analyzed using a generalized linear mixed model, feedforward neural network, and XGBoost. The study found that headache occurrences were associated with lower barometric pressure (p<0.001, gain=3.9) and significant decreases in barometric pressure (p<0.001, gain=11.7), higher barometric pressure at 6 a.m. (p<0.001, gain=4.6), higher humidity (p<0.001, gain=7.1), and increased rainfall (p<0.001, gain=3.1).
Further research is needed to enhance accuracy, ease of use, and generalizability, but the significant patient demand and industrial potential underscore the importance of this field.
ARTIFICIAL INTELLIGENCE APPLICATION IN RESEARCH OF HEADACHE DISORDERS
1. Basic research
Kogelman et al.66 collected temporal multi-omics profiles from 24 migraine patients during spontaneous migraine attacks, 2 hours after triptan treatment, during headache-free periods, and after a cold-pressor test. Relevant metabolites were evaluated using an ML method based on symbolic regression, QLattice.66 The study detected lower cortisol levels, higher sumatriptan levels, and elevated glutamine levels following treatment. Changes in sumatriptan levels were correlated with changes in GNA1 and VIPR2 gene expression, both of which are known to regulate cAMP levels.
Chiang et al.67 developed a DL model for the mouse grimace scale (MGS) called DeepMGS, utilizing the ResNet-18 architecture. This model automatically crops mouse face images, predicts action unit scores and total scores on the MGS, and infers the presence of pain. The system was tested on six migraine and six control mice, with performance compared to human scorers. The model achieved an accuracy of 70% to 90% and demonstrated a high correlation with human scorers in total MGS score (correlation coefficient=0.83).
Thomas et al.68 used a neural network model to replicate the neurophysiological dysfunction observed in migraine sufferers, specifically analyzing cortical-evoked potentials in response to repetitive visual and auditory stimuli. They developed normal and migraine synapse models for comparison. Upon repetitive presentation of stimuli at 40 dB and 70 dB input levels, the migraine model exhibited sensitization, with higher potentiating synapse strength resulting in a greater output.
2. Imaging
Hong et al.69 developed a system for the segmentation of deep white matter hyperintensities (WMHs) using a deep neural network based on the U-Net architecture. The model, applied to 148 migraine patients, comprised two networks: the first identified potential deep WMH candidates, and the second reduced false positives among these candidates. The models achieved a true positive rate of 0.88, a false discovery rate of 0.13, and an F1 score of 0.88 for segmenting deep WMHs.
CONCLUSIONS AND FUTURE PERSPECTIVES
The application of AI in the field of headache disorders is on the rise and has shown promising results. However, significant challenges remain in improving accuracy, generalizability and validation, ease of application, and linking findings to clinical relevance. Further research is needed in areas such as digital twins, which have been suggested as a potential tool in migraine management but have yet to be thoroughly explored.70
The appropriate use of AI holds great potential to enhance diagnosis, treatment, and research processes in the headache field. However, it is important to recognize that DL, ML, and various supervised and unsupervised methods do not always produce optimal results. No single approach—whether ML, DL, or supervised/unsupervised methods—is inherently superior to the other. Therefore, selecting the most appropriate method with careful consideration of study design is recommended. Caution is necessary when interpreting results, particularly with generative AI models such as LLMs.
Notes
AVAILABILITY OF DATA AND MATERIAL
The data presented in this study are available upon reasonable request from the corresponding author.
AUTHOR CONTRIBUTIONS
Conceptualization: WL, MKC; Data curation: WL, MKC; Formal analysis: WL, MKC; Investigation: WL, MKC; Methodology: WL; Writing–original draft: WL; Writing–review & editing: WL, MKC.
CONFLICT OF INTEREST
Wonwoo Lee was involved as a site investigator in a multicenter trial sponsored by Eli Lilly and Co., WhanIn Pharm Co. Ltd., and Handok-Teva. He has received lecture honoraria from Abbott and SK chemical in the past 24 months. Min Kyung Chu was a site investigator for a multicenter trial sponsored by Allergan Korea, Biohaven Pharmaceuticals, and Lundbeck Korea. He has received lecture honoraria from Allergan Korea, Handok-Teva, Eli Lilly and Company, and Yuyu Pharmaceutical Company in the past 24 months. Additionally, he received grants from Yonsei University College of Medicine (6-2021-0229), the Korea Health Industry Development Institute (KHIDI) (HV22C0106), and National Research Foundation of Korea (2022R1A2C1091767).
FUNDING STATEMENT
Not applicable.
ACKNOWLEDGMENTS
Grammatical error revision was supported by ChatGPT-4o.